Artificial intelligence (AI) is swiftly revolutionizing industries such as healthcare, finance, manufacturing, and transportation. However, this rapid expansion introduces a new challenge—protecting AI applications from cyberattacks.
The Expanding Threat Landscape:
IBM Security Report (2023): A staggering statistic from the 2023 IBM Security report estimates that the global cost of cybercrime will reach a whopping $10.5 trillion annually by 2025.
https://www.ibm.com/reports/data-breach.
Accenture Report (2020):
Adding to the concern, a 2020 Accenture report revealed that a significant 68% of AI leaders believe their organizations are vulnerable to AI-specific attacks
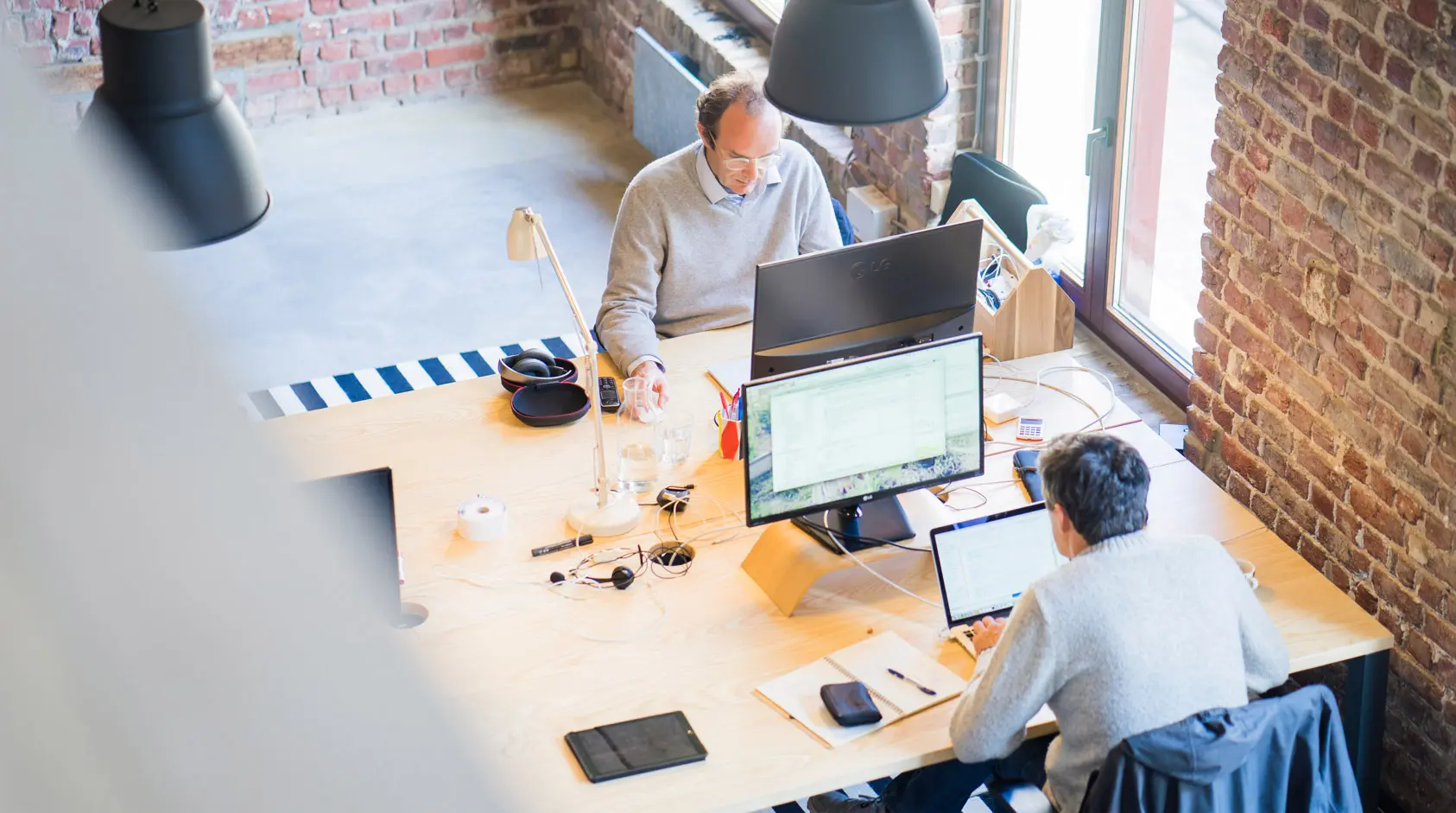
AndroxGh0st’s Expanding Arsenal of Exploits
These figures paint a concerning picture. AI applications are susceptible to various attack vectors, including:
Data Poisoning:
Malicious actors can inject poisoned data into training datasets, causing the AI to make biased or erroneous decisions.
Imagine training a spam filter to identify junk emails. A malicious actor could sneak in emails labeled as “important” but containing phishing links. Over time, the filter might learn to categorize even real phishing attempts as important, potentially causing financial losses.
Model Extraction:
Hackers might steal trained AI models, giving them access to the intellectual property and decision-making capabilities embedded within them.
Think of a self-driving car’s AI model that has been trained on millions of miles of driving data. Stealing this model would give someone access to the car’s “knowledge” of how to navigate roads, potentially allowing them to exploit weaknesses or create autonomous vehicles with malicious intent.
Adversarial Attacks:
These involve crafting specific inputs to manipulate an AI model’s output, potentially leading to safety hazards or financial losses.
Have you seen those pictures where a tiny change, like adding a sticker to a stop sign, makes a self-driving car misinterpret it? That’s a simplified example of an adversarial attack. Attackers can create specially crafted inputs, like slightly modified images, to trick an AI model into making wrong decisions. This could have serious consequences, like causing a self-driving car to miss a stop sign or a facial recognition system to misidentify a criminal.
Global Information Security Solutions for AI:
Data Security: Implementing robust data governance practices to ensure data integrity and prevent poisoning attacks. This includes data encryption, access controls, and regular data quality checks.
Model Security: Develop secure model development lifecycles with techniques like differential privacy and federated learning to protect model training data and prevent unauthorized extraction.
Threat Detection & Response: Utilizing AI-powered security solutions to continuously monitor AI systems for anomalies, identify potential attacks, and automate responses.
A Multi-Pronged Approach to AI Cybersecurity
The fight against cyberattacks on AI necessitates a unified effort from various stakeholders. Here’s a deeper dive into how different parties can contribute:
AI Developers:
Security shouldn’t be an afterthought. Developers should weave security considerations into the very fabric of AI development, from the initial data collection stage all the way to model deployment. This includes implementing techniques like differential privacy to anonymize training data, hardening systems against unauthorized access, and continuously monitoring models for signs of bias or drift.
For instance, an AI designed to recommend financial products could be vulnerable to bias if trained on a dataset that historically favored loans for certain demographics. Developers can mitigate this by employing fairness checks and incorporating diverse data sources during training.
AI Users:
Just like any powerful tool, AI requires responsible use. Users must be aware of the potential security risks associated with specific AI applications they employ. This involves understanding the limitations of the models and implementing robust security measures around them.
Imagine a company using facial recognition for access control. Security-conscious users would ensure the system is trained on a high-quality, unbiased dataset and implement two-factor authentication alongside facial recognition for added security.
Governments:
As AI continues to permeate various sectors, governments have a crucial role to play. They can develop and enforce regulations that promote responsible AI development and deployment, with a strong emphasis on security. These regulations could mandate security testing standards for AI models, require transparency in how AI decisions are made, and hold developers accountable for potential security breaches.
Security Researchers:
Staying ahead of the curve is paramount. Security researchers are the vanguard in this fight, continuously conducting research on AI vulnerabilities and developing new security solutions. Their work involves identifying novel attack vectors, creating tools to detect and mitigate threats, and fostering collaboration with developers to improve the overall resilience of AI systems.
For example, researchers might explore ways to detect adversarial attacks on self-driving cars or develop methods to prevent data poisoning attempts in AI healthcare systems.
Conclusion
By proactively addressing cyber threats, we can ensure the safe and secure implementation of AI. This will not only protect businesses and individuals but also unlock the full potential of this transformative technology. As AI continues to evolve, so too must our information security strategies. By working together, we can build a more secure and resilient future for AI.